Written by Antonios Saravanos, Clinical Associate Professor of Information Systems Management, School of Professional Studies, New York University. Antonios is also an Associate Research Fellow for the Cambridge Centre for Social Innovation and a graduate of the MSt in Social innovation.
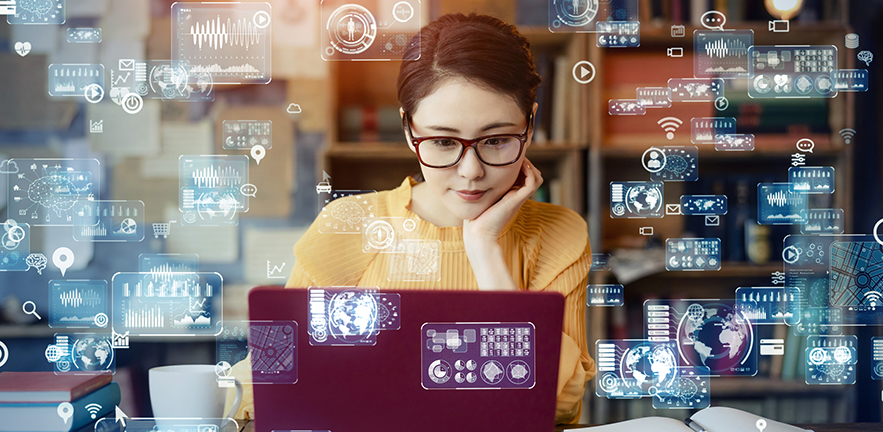
The online labour force: worth every penny?
Online services to facilitate participant recruitment for research have increased in popularity given their ability to lower the time, effort, and cost. One platform in particular which has emerged as a favourite is that of Amazon Mechanical Turk, colloquially referred to as Mechanical Turk or simply as MTurk (Goodman, Cryder and Cheema, 2013). In essence, “an online labour market” where “individuals and organisations (requestors)” are able to “hire humans (workers) to complete various computer-based tasks”, which they describe as “Human Intelligence Tasks or HITs” (Levay, Freese and Druckman, 2016).
The MTurk platform has been studied extensively, and the majority of publications report it to be suitable for recruitment (for example, see: Paolacci, Chandler and Ipeirotis, 2010; Casler, Bickel and Hackett, 2013; Crump, McDonnell and Gureckis, 2013; Samuel, 2018). I draw attention to Casler, Bickel, and Hackett (2013), who found that the quality of the data collected from crowd-sourced recruits is similar to that collected from in-lab participants and social media. Moreover, results have been found to be replicable (Rand, 2012), demonstrating the reliability of data (Paolacci, Chandler and Ipeirotis, 2010). Thus, data collected through MTurk is robust. Certainly, several studies highlight advantages over more traditional recruiting approaches, for example, increased sample diversity (Casler, Bickel and Hackett, 2013), participants exhibiting a higher level of attention (Hauser and Schwarz, 2016), and so on.
A focused workforce
Yet, a few studies trumpet warnings and reservations associated with the use of the platform (Chandler, Mueller and Paolacci, 2014). In ‘The Hidden Cost of Using Amazon Mechanical Turk for Research’ (Saravanos et al., 2021), we examined one such concern, the level of attention invested by a participant, focusing specifically on the most elite of available workers. Albeit, inattentiveness in participants, is not something new or unique to MTurk, but it is a bit unexpected given the context, as participants are at the end of the day workers. Accordingly, there is an expectation that workers would be fully attentive to the test task at hand. In particular, we looked at workers classified as “Master”, who had completed at least 98% of the tasks they committed to completing (i.e., an “Approval Rate” of 98% or greater). Additionally, these activities had to number above 999 (i.e., the “Number of HITS approved” had a value of 1000 or greater).
To control for any effects that compensation may have on participant attention, we offered a high level of compensation (> $22/hour). This was to address Paolacci Chandler and Ipeirotis (2010), who highlight that “given that Mechanical Turk workers are paid so little, one may wonder if they take experiments seriously“. To get an understanding of the magnitude of our compensation, Horton and Chilton (Horton and Chilton, 2010) reported that in 2010 that the mean wage was at $3.63/hr, and the median wage was $1.38/hour. However, Litman, Robinson, and Rosenzweig (2015) write that “a number of studies have examined how payment affects data quality” and “payment rates have virtually no detectable influence on data quality“.
Attention, attention
To investigate participant attention, we undertook an experiment where participants sourced through MTurk who met the criteria mentioned earlier were asked questions on their intent to adopt one of four hypothetical technology products. Three forms of attention check derived from the work of Abbey and Meloy (2017) were used to gauge participant attention. The first was a logical check which presented participants with two logical statements to answer. The second was an honesty check, where participants were asked two questions regarding their perception of the attention invested in the experiment. Each of the questions was rated on a seven-point Likert scale, ranging from “strongly disagree” to “strongly agree”. Participants who did not respond to both questions by selecting the “strongly agree” choice were deemed as having failed their respective attention checks. The third was a time check, disqualifying participants who were unable to complete the experiment within a reasonable period of time.
A total of 564 individuals took part in the experiment; from those, we found that 126 failed at least one form of attention check. The attention check that most (94) participants failed was the honesty check, followed by the logic check (31 participants) and the time check (27 participants). Several participants failed more than one check, with 14 participants failing both the logic and honesty checks and six participants failing both the time and honesty checks. Lastly, six participants failed all three of the attention checks (logic, honesty, and time). The number of participants who failed and passed all of these attention check forms are illustrated in Figure 1.
Spearman’s Rho correlations were generated to assess the relationship between the three different forms of attention checks. This experiment revealed positive correlations between participants who passed the logic and honesty checks (rs=0.310, p=0.000), those who failed the time and honesty checks (rs=0.132, p=0.002), and those who failed the time and logic checks (rs=0.139, p=0.001). In other words, the amount of time participants spent on the task was correlated to their passing the other attention checks (logic and honesty). Furthermore, participants who passed one of the three attention checks are more likely to pass the other two attention checks. We also examined the conjecture that perhaps that was a characteristic that might be linked to failing inattentiveness. Subsequently, logistic regression was used to investigate whether age, income, gender, marital status, race, and schooling had no statistically significant effect.
The takeaway from these findings are that: (1) high compensation does not eliminate inattentiveness; (2) a substantial (in our case 22.34%) of the most elite of workers on the platform are inattentive; (3) characteristics of age, gender, income, marital status, race and schooling cannot be used to predict inattentive behavior. Consequently, there is no simple solution to eradicate inattentiveness on the part of the participant. Rather to address this phenomenon of participant inattentiveness, researchers should contemplate adjusting their approach to account for the associated effort and costs required to identify those participants who are not paying attention and address their impact on the research.
References
Abbey, J. and Meloy, M. (2017) ‘Attention by design: Using attention checks to detect inattentive respondents and improve data quality’, Journal of Operations Management, 53–56, pp. 63–70. doi: 10.1016/j.jom.2017.06.001.
Casler, K., Bickel, L. and Hackett, E. (2013) ‘Separate but equal? A comparison of participants and data gathered via Amazon’s MTurk, social media, and face-to-face behavioral testing’, Computers in Human Behavior, 29(6), pp. 2156–2160. doi: 10.1016/j.chb.2013.05.009.
Chandler, J., Mueller, P. and Paolacci, G. (2014) ‘Nonnaïveté among Amazon Mechanical Turk workers: Consequences and solutions for behavioral researchers’, Behavior Research Methods, 46(1), pp. 112–130. doi: 10.3758/s13428-013-0365-7.
Crump, M. J. C., McDonnell, J. V. and Gureckis, T. M. (2013) ‘Evaluating Amazon’s Mechanical Turk as a tool for experimental behavioral research’, PloS One, 8(3), pp. 1–18. doi: 10.1371/journal.pone.0057410.
Goodman, J. K., Cryder, C. E. and Cheema, A. (2013) ‘Data collection in a flat world: The strengths and weaknesses of Mechanical Turk samples’, Journal of Behavioral Decision Making, 26(3), pp. 213–224. doi: 10.1002/bdm.1753.
Hauser, D. J. and Schwarz, N. (2016) ‘Attentive Turkers: MTurk participants perform better on online attention checks than do subject pool participants’, Behavior Research Methods, 48(1), pp. 400–407. doi: 10.3758/s13428-015-0578-z.
Horton, J. J. and Chilton, L. B. (2010) ‘The labor economics of paid crowdsourcing’, in Proceedings of the 11th ACM Conference on Electronic Commerce. Cambridge, Massachusetts, USA: ACM Inc., pp. 209–218. doi: 10.1145/1807342.1807376.
Levay, K. E., Freese, J. and Druckman, J. N. (2016) ‘The demographic and political composition of Mechanical Turk samples’, SAGE Open, 6(1). doi: 10.1177/2158244016636433.
Litman, L., Robinson, J. and Rosenzweig, C. (2015) ‘The relationship between motivation, monetary compensation, and data quality among US- and India-based workers on Mechanical Turk’, Behavior Research Methods, 47(2), pp. 519–528. doi: 10.3758/s13428-014-0483-x.
Paolacci, G., Chandler, J. and Ipeirotis, P. (2010) ‘Running experiments on Amazon Mechanical Turk’, Judgment and Decision Making, 5(5), pp. 411–419.
Rand, D. G. (2012) ‘The promise of Mechanical Turk: How online labor markets can help theorists run behavioral experiments’, Journal of Theoretical Biology, 299, pp. 172–179. doi: 10.1016/j.jtbi.2011.03.004.
Samuel, A. (2018) ‘Amazon’s Mechanical Turk has reinvented research’, JSTOR Daily, 15 May. Available at: https://daily.jstor.org/amazons-mechanical-turk-has-reinvented-research/ (Accessed: 20 October 2019).
Saravanos, A. et al. (2021) ‘The Hidden Cost of Using Amazon Mechanical Turk for Research’, arXiv:2101.04459 [cs]. Available at: http://arxiv.org/abs/2101.04459 (Accessed: 16 April 2021).
Leave a Reply